How An Early Warning System Can Leverage Data and Help Banks Effectively Manage Credit Risk
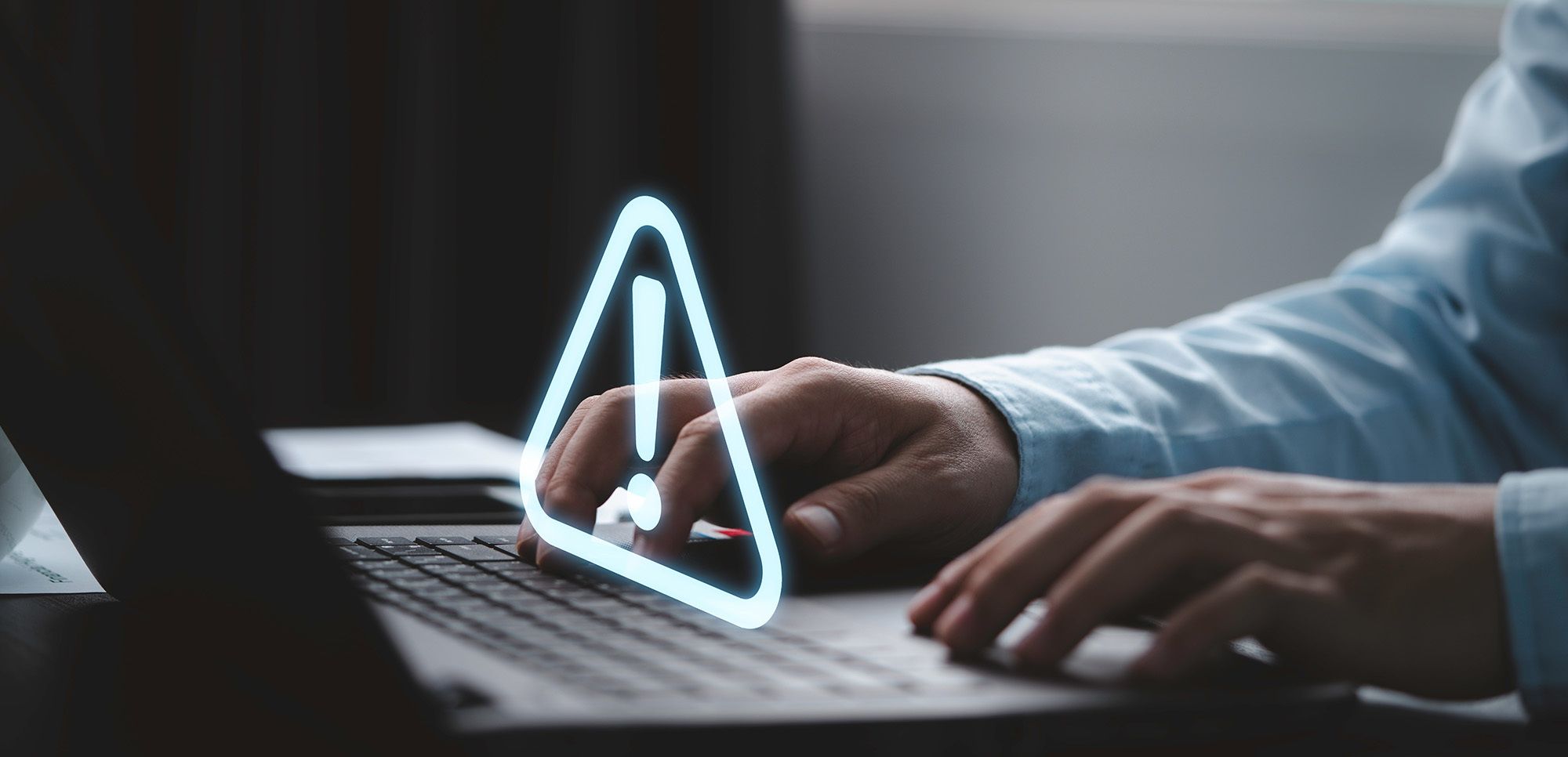
As per Reserve Bank of India’s (RBI) financial stability report, the Indian banking system encountered approximately 6,500 incidents of fraud worth ₹30,000 crores in the year 2019 alone. The lack of due diligence in loan disbursal and credit defaults are the primary contributing factors to these frauds. Add to this, the fact that the non-performing asset (NPA) ratio of all banks is expected to rise from 8.5% in March 2020 to 12.5% by March 2021, and with the new directive from RBI to increase lending to priority sectors like agriculture, SME, housing etc, it surely is going to be tough terrain for the banking industry.
Clearly, there is an urgent need for banks to reconsider their existing credit risk monitoring practices and switch from a compliance-driven reactive process to a proactive monitoring framework that is completely focused on predicting and preempting defaults well in advance so that banks can reduce their losses.
India’s chief economic advisor, Krishnamurthy V Subramanian too emphasized on the disruptive power of analytics for the banking sector. “Indian banking sector needs to invest in technology and data analytics to figure out the right model for large corporate lending which would in turn spur investment and help the economy grow into a $5 trillion one. If Indian banks used data analytics in times of corporate lending, many defaults could have been avoided”, he said.
It is imperative for banks to have an automated solution to track the credit health of borrowers. An Early Warning System(EWS) that is powered by Artificial Intelligence (AI) and Machine Learning (ML) will work 24×7 and can keep a live track on events that are leading indicators of distress.
An Early Warning System: Overview and Benefits
An early warning system is a set of guided processes that helps in identifying risks at an infant stage. An AI-powered EWS integrates data from a multitude of sources, from both public domains as well as from within the bank. With the help of this data and algorithmic intelligence, different indicators are projected that can help banks detect early warning signs of possible financial distress. A well designed EWS can help banks is not just effective credit risk monitoring but also serve the related purposes of fraud control and regulatory compliance.
Such a system can significantly lower the bank’s loan-loss contingency. With relevant EWS, banks can keep tighter control over their assets by:
A) Minimizing the probability of customer defaults:
Evaluating customer portfolios from time to time helps the banks in keeping a check on asset quality. The EWS systematically scans a customer’s portfolio, computes the relevant indicators and triggers appropriate action in case of a change in key indicators thereby catching signs of distress earlier than manual methods of occasional monitoring.
B) Securitization of assets:
Banks can attempt to securitize and sell assets where EWS indicators show low chances of recovery. This would help banks recover their money (to some extent) and get bad loans off their books
C) Identification of business patterns and opportunities:
AI-based EWS can also keep a track on the latest developments in various sectors and banks can accordingly change their portfolio mix. They can increase loan-disbursal to sectors that are more likely to grow and steadily reduce their exposure to sectors turning adverse.
Early Warning Indicators
The use of AI can enable EWS to mine early warning signals from this data. The early warning indicators of problem loans span financial, behavioral, geographic, and industry categories.
Financial Indicators
Financial indicators are among the most reliable ways to find out any asset-related issues and detect red flags pertaining to financial discrepancies and gaps. Financial indicators can also point out other financial problems, such as late loan payment, breaching the limit of operating loans, a delayed or missed employee payroll or returned checks drawn on the customer’s account.
Common data points in financial indicators that EWS reads to detect stress, include
- Customer’s creditworthiness
- Operating loan utilisation
- Expense management
- Cash flow requirements
- Covenants & collateral tracking
- Account receivables and debtor ageing
Industry Indicators
To minimize the risk on corporate loans, banks usually take into consideration the future growth of a customer’s business. For this, several industry indicators are measured and analysed, such as:
- Industry growth rate
- Industry regulations
- Ability to control costs/rising input prices
- Emerging market trends
- Changing customer behaviours
By taking into consideration all the above industry indicators, the EWS creates a forecast model that displays the statistics regarding the company’s growth.
Geographical Indicators
Geographical indicators include data points related to financial trends, country risks, and provide insights on international creditworthiness of an institution. They also are trackers of cross-border transactions contributing valuable insights to anti-money laundering activities.
Geographic contributors to corporate credit risk, include:
- Exchange rate devaluation.
- The yield on sovereign bonds
- Money laundering activities
- Country risk due to changes in the political environment
- Economic growth in the region.
Behavioural Indicators
Two of the governing principles of banking are trust and credibility. When doling out large sums of money, especially to corporates, banks must take into consideration behavioural indicators that give insights about the integrity and competence of the personnel running the corporate.
Behavioural indicators for corporate credit risk include:
- Delayed financial reporting requirements
- Deception/misrepresentation of facts
- Lack of responses
- Providing irrelevant information
- Misrepresented or destroyed records
- Lack of stakeholder interest in business strategy and planning
- Too much change in accounting personnel or procedures.
- Creating fake or shell entities
Cadenz.ai – The Customer Data Platform for EWS
Cadenz, the market’s first customer intelligence platform, is an advanced form of Customer Data Platform (CDP) that provides ready-to-use technology for continuous monitoring of corporate risk.
Cadenz performs an in-depth risk assessment by collating data from multiple sources to provide intelligent insights along with a detailed view of the company’s financial profile that enables the analysis of credit risks and lending along with continuous risk monitoring.
Cadenz – Use Cases in Credit Intelligence
The business goal of a CDP is to bring the customer data together in one central location and analyze it to project indicators that can give banks early warning signals of financial distress if any. With Cadenz CDP, banks can improve their decisions, delivery speed, and their overall customer experience.
Written below are few use cases showcasing how banks are using Cadenz to improve their credit business and operations:
Use Case 1: SOE bank augments EWS with external intelligence
Given its deep market penetration, the SOE bank had a large portfolio of companies to monitor for risk. The bank’s early warning system (EWS) was primarily picking up patterns inferred from first-party data. Though RBI has prescribed tracking early warning indicators from second-party and third-party sources, the bank found it difficult to implement without scalable technology, accurate data and cutting-edge ML algorithms to derive insights on corporate behaviour from the data. Critical inputs on the viability of client projects and, at times, overall client business as well as the legitimacy of the end-use of loans were also missed out.
Cadenz Solution
Cadenz gathered various datasets pertaining to the company’s performance including compliance filings, news, legal, developments and capital market events. The data derived from second-party and third-party sources were incorporated into the EWS model insights. Cadenz also monitored companies continuously to check for changes in business-related and compliance KPIs.
Cadenz helped in increased accuracy & early detection of potential stress along with the reduction in operational costs due to automation in information processing. A pilot study on 100 corporate entities over 3 months identified 5% more cases with high-potential of defaults than when using only first-party data.
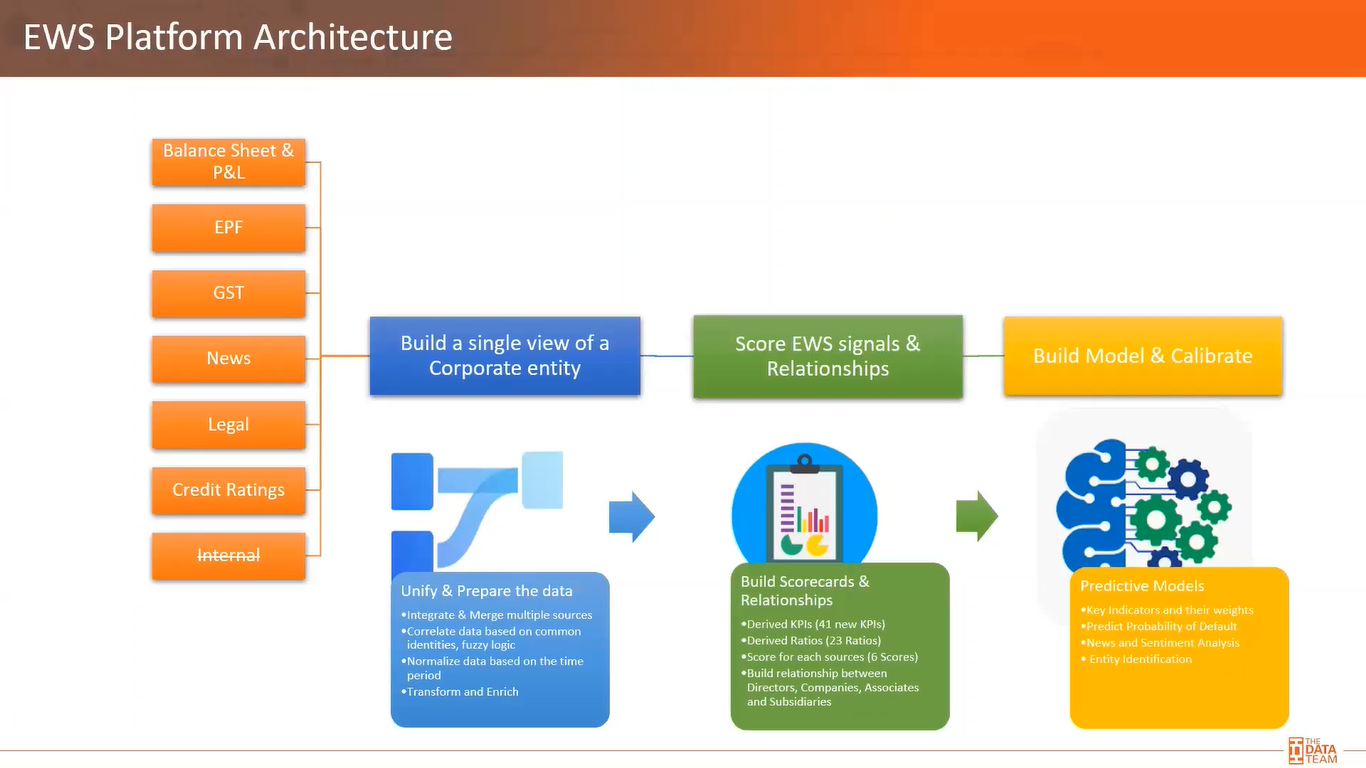
Use Case 2: Leading Bank Used Cadenz To Gauge Business Sentiment & Viability
As mentioned above, behavioural indicator is one of the most important early warning signs banks benefit from during risk monitoring. For a leading bank in India, Cadenz analysed over 6,334 news articles, snippets and releases from 28 different sources, including NSE company announcements between Oct 2019 – March 2020.
The news pieces and other press data were studied to gauge the viability of the customer sentiment as a lagging indicator. The relative sentiments from the news helped in detecting the probability of customer stress. The analysis helped detect that the average (of all sentiments of all the articles over a week) negative sentiment was among the top 15 attributes contributing to the probability of stress and is a significant indicator of nearly 2-4% of defaults.
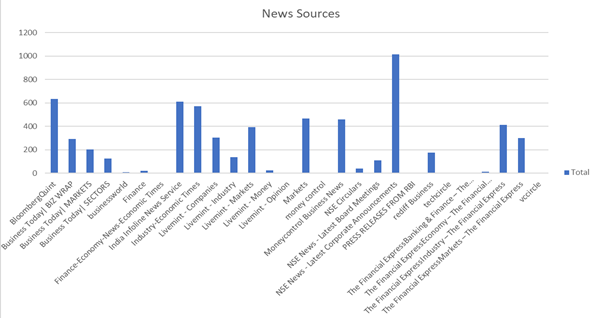
Use Case 3 – Cadenz Helped A Private Sector Bank Overcome Challenges With Flow-Based Lending & Monitoring
For most corporates, the practice of receiving credit from banking institutions is lengthy and time-consuming for both the parties involved. For the bank, the post-disbursal monitoring is challenging as business activity spans multiple data points that are hard for a human to assimilate and derive risk insights.
Cadenz helped a private sector bank extend easy and fast credit facilities to corporates with its ML-powered flow-based lending solution. The solution automatically appraised the use of existing loans, computed business behaviour metrics, and then scored the loan application.
Using Cadenz, the bank was able to shorten the entire credit approval process from an average of 5 days to just under 3 hours! Moreover, new business activity was continuously analyzed using the same flow-based models to keep refreshing up-to-date models of ongoing business-related risk.